Advances in teleophthalmology and artificial intelligence for diabetic retinopathy screening: a narrative review
Introduction
Diabetic retinopathy (DR) is the leading cause of preventable vision impairment in working-age adults. The International Diabetes Foundation projections estimate that 783 million people worldwide will have diabetes by 2045 (1). Currently, only 30–40% of patients with diabetes adhere to recommended diabetes screening guidelines (2). As the disease burden of DR increases, there is a growing need to screen patients and intervene early. Advances in remote retinal imaging and artificial intelligence (AI) have led to a surge in their use for diabetic eye screening (3). Teleophthalmology programs and AI algorithms are burgeoning, with the aim to improve research, teaching, and healthcare access. The Coronavirus disease 2019 (COVID-19) pandemic further increased awareness and interest in expanding remote eye care to allow continued interactions between providers and patients in a safe manner.
The goal of this review article is to present the literature about the current state of teleophthalmology and AI for DR screening. In this review, we discuss the various teleophthalmology programs, advances in remote imaging technologies, the use of machine learning in DR screening, and the legal aspects of remote and automated screening. We present this article in accordance with the Narrative Review reporting checklist (available at https://aes.amegroups.com/article/view/10.21037/aes-23-10/rc).
Methods
We conducted a literature review using PubMed and Cochrane (accessed January 2023) to identify articles involving teleophthalmology and AI for DR screening published between 1994 to 2022 using a combination of the following search terms:“teleophthalmology”, “teleretinal”, “telemedicine”, “retinal screening”, “artificial intelligence”, “machine learning”, “legal ramifications”, and “diabetic retinopathy” (Table 1).
Table 1
Items | Specification |
---|---|
Date of search | January 1, 2023 |
Databases and other sources searched | PubMed, Cochrane |
Search terms used | Diabetic retinopathy, eleophthalmology, teleretinal, telemedicine, retinal screening, artificial intelligence, machine learning, and legal ramifications |
Timeframe | 1994–2022 |
Inclusion and exclusion criteria | Inclusion criteria: publications about retinal screening services and the use of artificial intelligence in screening |
Exclusion criteria: non-English literature | |
Selection process | Conducted independently by all authors; discussed and reviewed prior to manuscript preparation |
Teleophthalmology for DR screening
The use of telemedicine in healthcare has been gaining traction in recent decades. Remote eye care using teleophthalmology can be applied for the evaluation of anterior segment diseases, retinopathy of prematurity, age-related macular degeneration (AMD), glaucoma, and DR (4). Remote DR screening programs often employ fundus imaging at a non-eye care setting, followed by evaluation of the images by an ophthalmologist, optometrist, and supervised trained grader which may then lead to a possible referral for in-person evaluation. Studies have shown that the sensitivity (62.5–98.2%) and specificity (76.6–98.7%) of teleophthalmology for detecting DR remotely are comparable to those of traditional in-person clinical examinations (5-7). Currently, there are several national and international DR screening programs that employ different fundus imaging technologies and workflow.
Remote DR screening programs
The classification of DR is defined by the Early Treatment for Diabetic Retinopathy Study (ETDRS), based on worsening severity of microvascular retinal changes from mild to moderate to severe nonproliferative DR (NPDR) and presence of retinal neovascularization in proliferative DR (PDR) (8). Diabetic macular edema (DME) and neovascularization are the two driving factors for vision loss in DR. The American Telemedicine Association (ATA) has specified four categories of validation for DR telehealth programs, depending on the clinical performance and goal of the program. A Category 1 program distinguishes none or very mild NPDR from mild to more severe DR for further evaluation. A Category 2 program differentiates between non-sight threating and sight-threatening DR (defined as DME, severe NPDR, or PDR). Category 3 can differentiate between more subgroups of DR to determine disease management, and Category 4 can match or exceed disease identification compared to the current gold standard of ETDRS photography (9).
Category 1 and 2 programs require minimal resources to initiate screening services and can screen large numbers of patients. EyePACS, a non-proprietary web-based DR screening tool, is a Category 1 program that services over 600 organizations with over 750,000 screened and has been able to access wider audiences such as the Mexican immigrant community (10). However, to maintain this screening tool at a prorated cost, clinics that use the EyePACS program must meet specified number of DR screenings performed per month, which is a potential challenge (11). EyeCheck is a Category 2, web-based, remote DR screening program developed in the Netherlands for primary care offices. Their workflow includes the primary care physician or staff capturing two-field retinal photography, with or without dilation based on the screening site, that is graded by two ophthalmologists. This program reported a high rate of inter-grader agreement (κ=0.93); however, the EyeCheck program has not been directly compared to ETDRS photography so far. This program was able to screen 30% of the diabetic population in the Netherlands by 2010 (12,13).
Category 3 programs provide more granular DR classifications. Joslin Vision Network (JVN) is a well-validated Category 3 program developed in 2000 in collaboration between the Joslin Diabetes Center and United States government entities, including the Veteran’s Affairs (VA) administration, Indian Health Service (IHS), the Department of Defense. Despite JVN using a slightly modified protocol with three stereo fields (Table 2) compared to the standard seven-field ETDRS protocol, moderate agreement (κ=0.65) was demonstrated between the two methods (22).
Table 2
ATA category (10,14) | Program name | Location of employment | Start year | Patient volume in pilot study | Referral rate for retinopathy in pilot study |
---|---|---|---|---|---|
Category 1 | Digiscope (15) | 51 PCP offices (in 7 states and the District of Columbia) | 2002 | 2,771 patients over 6 months | 19% |
EyePACS (16) | 120 primary care sites | 2003 | 3,562 encounters over 1 year | 8.21% | |
Ophdiat (17) | 16 screening centers in Île-de-France | 2004 | 15,307 DR screening examinations over 2 years | 25.2% | |
Category 2 | EyeCheck (13) | Family care physicians’ offices or family physician laboratories | 2003 | 1,676 patients over 1 year | 10% |
National Health Services diabetic eye screening (18) | General practices in Gloucestershire | 2003 | 3,661 patients over 2 years | 18.5% | |
Category 3 | University of Alberta (19) | General practitioners and community health representatives in Fort Vermilion | 1999 | 100 patients over 8 months | 10% |
Indian Health Service-Joslin Vision Network Teleophthalmology Program (20) | 80 IHS/tribal/urban primary care clinics in 24 states | 2000 | 1,291 patients over 1 year | 25.5% | |
RAMP (21) | Family medicine and general outpatient clinics in Hong Kong | 2010 | 174,532 diabetic subjects over close to 4 years | 9.8% |
ATA, American Telemedicine Association; PCP, primary care physician; DR, diabetic retinopathy; IHS, Indian Health Services; RAMP, Risk Assessment and Management Program.
A trained eye-specialist manually reads the images, via software and reports DR severity determination and recommended treatment. The JVN protocol has a sensitivity of 98% and specificity of 100% within one ETDRS grade among eyes and high agreement (κ=0.87) for referral recommendations for further evaluation. The JVN teleophthalmology program screened over 70,000 patients within the first ten years and has since provided more DR screening nationally. A notable strength of the JVN protocol is the educational component of their screening completed by a certified patient care coordinator; a survey of the participants showed that 96.1% agreed or strongly agreed that they had a better understanding of diabetes-related eye disease after their visit. JVN has partnered with both the VA and IHS to create an extensive database of patients to allow for a standardized screening process, while also improving eye care access for the vulnerable populations of Veterans and Native Americans, respectively. Both the VA and IHS conduct DR screenings using a protocol similar to the JVN screening protocol (10). The VA has performed over 120,000 examinations and a randomized study showed that patients at the VA were more likely to complete their annual teleophthalmology screening compared to their traditional mydriatic screening service (87% vs. 77%, P<0.01) (23,24). The VA Healthcare System’s initiative, Technology-based Eye Care Services (TECS), is operating in both urban and rural primary care clinics; their current trial results show 97.6% agreement in their TECS screening protocol-based detection results compared to that of face-to-face testing (25). Compared to the comprehensive eye clinic, an advantage of TECS screening is that both the patient and physician time spent was shorter by more than 25% and 50%, respectively, which allows for more patients to be evaluated (26). Through partnership with JVN, the IHS has set up over 99 sites within 25 states and has screened over 230,000 participants. The IHS-JVN is the largest Category 3 telemedicine program based in primary care settings and has subsequently experienced a significant improvement in DR screening rates at local, regional, and national levels. Within four years of initiation, the rate of annual retinal screening increased by 50% and the rate for laser photocoagulation treatment increased by 51% within this population (27). The University of Alberta has also set up a Category 3 telemedicine program for remote DR screening and management for diabetic patients in remote regions of Canada, as many patients reside over 600 miles from an ophthalmologist (19). Their workflow includes the capture of mydriatic digital photographs at a remote site, use of a proprietary web-based system that allows for image compression, and evaluation of images by an ophthalmologist who recommends treatment or future follow-up with an in-person eye exam. The Alberta protocol only uses two fields for imaging, but the protocol showed high accuracy (κ>0.71) when compared to seven-field ETDRS photography (28). This program has screened over 6,000 people, with over 1,000 patients referred for treatment. A strength of this program is that for patients who screen positive for vision-threatening DR (vtDR), the University of Alberta will arrange all the testing and treatment to be completed in a single visit, which is crucial for patients who live in remote locations with limited access to eye care services.
There has been an increased interest in expanding DR screening beyond primary care sites to include settings such as community centers, pharmacies, and student-run free clinics, as these locations serve as additional touchpoints for screening patients who miss their recommended annual eye exam. Gibson examined a group of US adults over two years and found that 51.9% of the sample missed their annual diabetes prevention visit with their primary care or eye care provider (29). In India, strong efforts to increase DR screening in more rural communities via community health centers face challenges due to the population density. Shukla et al. described a study comparing the number of participants reached via a fixed site at a community center compared to screening mobile vans equipped with fundus cameras (30). After screening over 8,000 patients, they found that the vans were able to reach more individuals with worse DR pathology/severe vision impairment. However, only 35.7% of patients who were screened came back the following year for their annual eye screening, which emphasizes the need for sustainable screening services in remote locations (30). Screening for DR has also been proposed at pharmacies in efforts to target wider audiences at every interface of patient and healthcare. Through a pilot survey used to evaluate the role of pharmacies in DR screening, Law et al. report that a majority of the responding pharmacy staff members find benefit in establishing DR screening in community pharmacies (31). However, there are several barriers to starting DR screening at pharmacies, namely the shortage of pharmacy staff and the cost it would take to purchase equipment and conduct screenings (31). Student-run clinics, which often serve as primary care centers for underserved and uninsured populations, have also become sites where DR screening can be completed. Figure 1 shows a typical fundus camera used for remote screening. Licensed providers and student volunteers have started implementing telemedicine within the scope of their care (32), however, only 21.7% of student-run clinics offer ophthalmology services nationally (33).
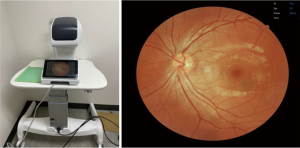
Advantages and challenges of teleophthalmology
Teleophthalmology can increase DR screening rates, enable earlier eye care access, and reduce healthcare costs (34). Enhancing eye care access is particularly valuable for patients who live in rural or underserved communities (35-37). The Los Angeles County’s teleophthalmology program has increased DR screening rates by 16.3% and eliminated a potential 14,000 referrals to eye centers (38). The English National Health Service has increased screening rates to over 82%, with over 2.5 million patients screened annually (39). Using national insurance claims data from the OptumLabs Data Warehouse, Lieng et al. also found that remote imaging provided a median time to DR screening of 2.0 months, as compared to 3.4 months for in-person visits after initial diagnosis of type 2 diabetes mellitus, suggesting that telemedicine enabled earlier eye care access for patients with newly diagnosed diabetes (40). Finally, teleophthalmology can lower healthcare costs by reducing the need for in-person ophthalmology visits.
A major barrier to widespread adoption of teleretinal screening is financial sustainability. Beyond the upfront cost of purchasing fundus cameras and equipment maintenance, these programs also incur indirect costs associated with the time for medical personnel to operate these devices and for eyecare providers to interpret the images. Despite the surge in teleophthalmology utilization over the past decade, insurance coverage has dwindled, with proportion of claims paid to physicians decreasing from 88% in 2011 to 47% in 2020 (41). Additionally, for claims that were paid, mean inflation-adjusted payments for teleophthalmology CPT codes 92227 and 92228 remained relatively stagnant from $12.85 and $19.31 in 2011 to $14.85 and $25.10 in 2020, respectively, as compared to payments for in-person fundus photography 92250 which increased from $45.15 to $64.70 over the same 10-year period (41). At a large integrated health system in Northern California, insurance coverage for remote DR screening using telemedicine CPT codes was also found to be highly inconsistent, with only 44.7% of charges paid and a majority of denials coming from Medicare as a non-covered benefit (42). In fact, this cost analysis showed that direct payments for teleophthalmology would not be financially sustainable without taking into account quality incentive bonuses and downstream revenue from in-person ophthalmology referrals within the same care network (42). In March 2020, during the initial period of the COVID-19 pandemic, which was declared a public health emergency, the Center for Medicare Services (CMS) expanded guidelines for telehealth reimbursements under Section 1135 of the Social Security Act. Although this led to an increase in insurance coverage for teleophthalmology CPT codes in early 2020, payment rates largely returned to pre-pandemic levels by the end of that year (43). Thus, despite clear clinical and financial benefits from a public health perspective, the current landscape of insurance payors limits the fiscal sustainability of remote DR screening programs outside of single-payor systems such as the VA or Kaiser Permanente.
Another barrier is the task of integrating teleretinal imaging into primary care settings, as adding a new service can pose significant work-flow challenges. Using a qualitative approach interviewing healthcare personnel involved with incorporating telehealth for DR screening at Federally Qualified Health Centers (FQHCs) in California, medical staff reported increased strain on personnel due to difficulties scheduling patients, tracking referrals, and limited resources for educating and communicating with patients (11). In many cases, staff had to create workarounds outside of their job descriptions or usual workflow, which is not a sustainable model of healthcare delivery. Examples of these workarounds include medical assistants who ensured appropriate screening by scheduling screening visits for patients with elevated HbA1c, as a workaround for those without a diabetes diagnosis code on their record. Additionally, ophthalmologists included diabetes management education into their eye appointment and sent retinal images via their personal phones to increase patient disease understanding and treatment adherence. Notably, a limitation of this discussion of advantages and challenges is the reporting of findings within the United States; the field of teleophthalmology in Europe and other countries faces unique challenges that require separate consideration.
Advances in remote imaging technologies
Despite the various challenges limiting widespread adoption of teleophthalmology, recent advances in fundus cameras, electronic health record (EHR) integration, and remote work continue to generate interests in expanding teleretinal screening. Compared to older models of fundus cameras that were expensive, complex, and often require a trained ophthalmic photographer to operate, newer generations of cameras are cheaper, easier to use, and can automatically locate and focus on the subject’s retina with relative ease (44). Most devices can be operated by a nurse or medical assistant with little training (Figure 1). More portable fundus camera designs, including some that incorporate smartphones to provide the processing power, significantly reduce the physical footprint and costs associated with tabletop fundus cameras (45). Although these low-cost devices may not provide the same high-quality retinal images as conventional fundus cameras, with some devices reporting high specificity (77–99%) and variable sensitivity (6–77%) in detecting different retinal pathologies, many of them may be adequate in settings where eyecare access may otherwise be limited (46). Use of a handheld non-mydriatic retinal camera in a resource-limited setting with high prevalence of diabetes captured images of sufficient quality for DR screening in the Pacific Island of Vanuatu (47). A meta-analysis to assess the diagnostic accuracy of smartphone-based retinal imaging technologies for detecting DR found that these devices performed well in detecting DR (pooled sensitivity of 87% and specificity of 94% for detecting any DR), with the highest diagnostic accuracy for late stage and treatment-requiring DR (48).
Beyond the choice of fundus cameras, teleophthalmology programs differ in the imaging protocol used for DR screening (14). Compared to the traditional ETDRS protocol, most current fundus cameras have larger fields of view requiring only two or three images to be captured. Three fundus fields with nonmydriatic color 45-degree photography have been shown to be as effective as standard ETDRS protocol for detecting referable levels of DR (sensitivity of 82% and specificity of 92%), while one fundus field photography has the capacity to determine absence or presence of DR (sensitivity of 71% and specificity of 96%) but does not distinguish DR severity levels (49). Ultra-wide field (UWF) cameras that employ scanning laser technologies are more expensive but can capture up to 200-degree field of view in one image. A comparison of UWF with ETDRS imaging demonstrated exact agreement in DR severity in 59.0% of the eyes and agreement within one DR severity step in 96.9% of the eyes evaluated by both imaging modalities (50). Ashraf et al. found exact agreement in 87% of eyes and agreement within one DR severity step in 99.1% of eyes evaluated by 4-field 60° × 55° stereoscopic widefield imaging compared to ETDRS field area (51). In fact, UWF imaging identified more severe DR in 10% of subjects (52), reduced the rate of ungradable images by 71%, and identified DR more frequently by 17% (53). Thus, despite the higher cost, newer UWF imaging technologies may provide greater sensitivity than standard fundus cameras.
Additional research studies focus on the optimal number of images and the use of dilation with imaging (13,54,55). Although the seven-field ETDRS protocol is the gold standard for determining DR severity, adding more image fields to two 45-degree images did not significantly increase sensitivity or specificity of DR screening (54). The use of pupillary dilation has also been debated, as pharmacologic dilation is burdensome and may pose some risks, especially to patients with narrow angles. The EyeCheck program recommended a ‘dilate-as-needed’ approach to retinal imaging, as only 11.3% of participants required pupillary dilation (13). A meta-analysis by Bragge et al. reported that variations in mydriatic status alone did not significantly influence sensitivity (odds ratio: 0.89, P=0.61) or specificity (odds ratio: 0.94, P=0.80) to detect any DR (55).
Machine learning in DR screening
The use of AI has the potential to vastly improve the efficiency, cost, and sensitivity for DR screening. Advances in machine learning algorithms, particularly those incorporating deep learning, can provide instantaneous result feedback, handle large volumes of images without fatigue, and enable greater sensitivity and accuracy for determining DR presence and severity than their human counterparts (Table 3).
Table 3
AI name | Sensitivity/specificity | FDA approval |
---|---|---|
IDx-DR | 96.8%/87% (56-59) | FDA-approved in USA |
EyeArt | 99%/80% (60) | Approved in EU, commercially available in Canada |
EyRis SELENA+ | 92.25%/89.04% (61) | Approved in EU and Singapore |
Google/Verily | 97%/96% (62) | Not yet FDA-approved or commercially available |
AI, artificial intelligence; FDA, Food and Drug Administration.
In 2016, researchers from Google AI showed that a deep convolutional neural network trained to grade DR and DME from fundus images could achieve a sensitivity and specificity of up to 97.5% and 96.1%, respectively (62). The algorithm performed better than retina specialists, who only achieved 93.5% sensitivity and 84.8% specificity (63). The use of deep learning reduced false negative rates by 23% and increased false positive rates by 2% (63). Combining with available modifiable and non-modifiable risk factors such as hyperglycemia, hypertension, dyslipidemia, access to care, and duration of diabetes allowed the algorithm to predict the prognosis for DR risk at 2 years and beyond (64).
In 2017, Abràmoff and colleagues reported results of the first pivotal trial to assess the clinical safety of autonomous AI for screening referable DR (56). With over 900 adult diabetic patients spanning 10 primary care sites in the U.S., the IDx-DR (Digital Diagnostics) system was able to distinguish referable from non-referable DR, where referable DR was defined as more than mild NPDR (mtmDR) (56,65). Sensitivity results using IDx-DR ranged between 87–98% and in later studies showed no false negative results when compared to expert raters (56-59). These figures were not significantly affected by race or ethnicity (66). In 2018, IDx-DR became the first Food and Drug Administration (FDA)-approved autonomous AI software for any field in medicine (56). In the same year, IDx-DR was also validated in the Dutch healthcare system, achieving a sensitivity/specificity of 91%/84% and 68%/86% using the EURODIAB and ICDR grading standards, respectively (59). The authors speculate that the lower performance may be due to classification of a single hemorrhage as more than mild DR under the ICDR scale. Currently, the platform is approved for use with the Topcon NW400 non-mydriatic fundus camera only, limited to detecting only DR and macular edema, and not recommended for use for any other disease or condition.
The approval of IDx-DR paved the way to a series of other automated DR detection platforms, such as the EyeArt system, which received clearance from the FDA in 2020 and detects both mtmDR and vtDR, defined as severe NPDR, PDR, or clinically significant DME. The automated platform was similarly found to be comparable to grading by certified ophthalmologists and cost (67-69). The pivotal trial enrolled 893 diabetic patients across 15 U.S. study centers to test the platform’s ability to discriminate mtmDR or vtDR from 2-field undilated fundus photographs. The system demonstrated 95.5% and 95.1% sensitivity, and 85.0% and 89.0% specificity for mtmDR and vtDR, respectively (70). EyeArt has also been adapted for smartphone-based fundus camera images. Even though the algorithm was not specifically trained on mobile images, it still achieved a sensitivity/specificity of 99%/80% for sight-threatening DR (60), despite the limited field of view (71).
Despite the cost effectiveness of fundus cameras, more advanced laser-based imaging technologies such as optical coherence tomography (OCT) enables greater sensitivity to detecting macular edema, as well as other conditions such as AMD and glaucoma. Deep learning enables prediction of OCT-derived DME grades from fundus photographs, with higher specificity and equal or greater sensitivity when compared to human graders (72-76). Machine learning networks can also detect other diseases from OCT such as central serous chorioretinopathy (77) retinoschisis, macular hole, retinal detachment, and myopic choroidal neovascularization (78). More recently, the EyRis SELENA+ deep learning platform, which is designed to simultaneously detect DR, AMD, and glaucoma from fundus images have been implemented in a national screening program in Singapore (79,80). Tested as part of a screening mobile unit in Zambia, SELENA+ demonstrated a sensitivity/specificity of 92%/89% for referrable DR (61). Thus, the incorporation of additional imaging modalities is not only improving the accuracy of disease detection, but also detection of multiple common eye diseases.
Implications and limitations of automated screening
The incorporation of AI into DR screening can improve cost-effectiveness and close the care gap for remote DR screening, while also improving the sensitivity and specificity of disease detection (56,57,59), in some cases exceeding the accuracy of human graders (73,81-84). Without the additional work-flow of sending images to a human grader and the turnaround time for receiving a report, automated systems enable instant feedback, allowing primary providers to discuss the results with the patients at the same visit and immediately referring them to an ophthalmologist if necessary. However, the incorporation of AI into clinical practice also raises concerns over privacy and data security, transparency around the “black box” in AI, finances, and the ability to detect other potential sight-threatening ophthalmic conditions.
Use of an AI system introduces the challenge of understanding and trusting the “black box” by both providers and patients (85-89). There are also concerns on the possibility of inequitable downstream recommendations if AI systems are trained on biased data (90). Transparency in the process utilized for arriving at a particular diagnosis or decision to refer is necessary for developing trust and maintaining or improving patient-centered outcomes (86,87). An ongoing effort in the field is ‘explainable AI’ (XAI) that focuses on increasing the users understanding by offering precise explanations for AI-generated output. By contextualizing the results, XAI provides more transparency and increases the users trust and understanding in the predictions (91). Many automated platforms require sending de-identified images to cloud-based servers. Although retinal images are not traditionally considered protected health information (PHI) based on the Health Insurance Portability and Accountability Act (HIPAA), the increasing reliance on fundus images as biometric identifiers is limiting the ability to safely share retinal photographs for automated DR detection. Additionally, research suggests that machine learning may be employed not only to detect disease, but also to determine age, sex, refractive error, blood pressure, hemoglobin A1c, and other systemic cardiovascular conditions (92-96) from fundus photographs, with broad implications for patient privacy and the types of health information that can be shared (92). Clinicians and patients need assurance that these systems can update predictions based on new data (87,97). Some have suggested the need for both clinician education and consolidation of datasets in order to increase trust (86,98). Finally, there are concerns regarding health equity from the implementation of AI systems in resource-scarce areas. One must carefully consider the availability of follow-up care after screening to optimize patient outcomes and cost savings (61,99).
The finances of implementing AI for teleophthalmology has not yet been clearly determined. Business models may include a monthly subscription service for the cameras and/or software, or a pay-per-photo plan, both of which benefit from high patient flow through centralized locations where the retinal cameras can be effectively deployed as point-of-care testing sites. Additionally, many algorithms are approved for only a specific retinal fundus camera, so clinics that already invested in upfront capital of purchasing an older camera model may need to purchase additional new equipment rather than leveraging existing instruments. In 2021, CMS developed a new CPT code 92229 for AI-based teleretinal imaging, but reimbursements for 92229 varies with region and payor mix, and do not include a physician fee since only a technical component is billed. For example, Cigna Government Services (CGS) priced the use of AI-enabled screening at $28.42 compared to regions (Florida, Puerto Rico, US Virgin Islands) covered by First Coast Service Options priced at $54.51 (100). Although First Coast covers states that have higher prevalence of diabetes compared to Kentucky, carriers in these regions pay double that of CGS’s carriers (100).
Importantly, while AI-based DR screening is accurate and effective, many of these systems are not designed (or at least approved) to detect non-DR pathologies like AMD and glaucoma. In contrast to an ophthalmologist or optometrist who have the ability to diagnose a variety of ophthalmic conditions, supervised machine learning algorithms are only able to identify pathologies that they are trained to detect. While unsupervised deep learning methods are still in their infancy, some platforms such as the SELENA+ can detect multiple conditions at the same time (57,61,101). Unsupervised or self-supervised deep learning has the potential to identify novel ocular pathologies without training with explicitly-labeled data, and could represent the future of AI in teleretinal imaging (102).
Legal aspects of remote and automated screening
An important consideration of advancements in teleophthalmology and AI is the ethical and legal impact of new technology and workflow. Government regulations have not kept pace with advances in telemedicine (103), with only limited legislature passed addressing medical licensing, professional liability, accreditation, online prescription, informed consent/data security, and definition of the patient-doctor relationship in telehealth (104,105). For licensure and liability, the Federation of State Medical Boards approved the Interstate Medical Licensure Compact in 2014, which accelerates the licensure process to allow physicians to provide care in other states (105). For online prescriptions, The Ryan Haight Online Pharmacy Consumer Protection Act of 2008 aims to prevent illegal distribution of medication via the Internet. Data confidentiality continues to be addressed via the HIPAA (106). During the COVID-19 pandemic, additional legislature was put into action—for example The Coronavirus Aid, Relief, and Economic Security (CARES) Act, that promoted the expansion of telemedicine (107). Ongoing global efforts are underway to regulate and standardize telemedicine care.
Despite the promise of AI in improving healthcare, concerns around transparency, misdiagnosis, exacerbation of socioeconomic biases, and patient privacy must be considered. There are limited regulations that address the use of AI in medicine (108). Most regulations exist as drafts, guidelines, or government initiatives. In 2018 the American Medical Association (AMA) adopted the Augmented Intelligence in Healthcare Policy (H-480.940) to ensure an emphasis on the ethical evolution, education, and AI in clinical practice (109). This policy is based on two main criteria—first, AI should be used only as a tool to aid clinical judgement rather than to replace it, and second, tool development must have clinically validated and ethically sensitive designs to safeguard patient privacy and safety (110). Additionally, the US FDA has approved AI tools for various clinical uses outside of ophthalmology such as stroke diagnosis, brain magnetic resonance imaging (MRI) interpretation, and atrial fibrillation detection, among other applications (111). The impact or sufficiency of these policies remains unclear.
Conclusions
The use of teleophthalmology and AI algorithms expands eye care access and helps prevent vision loss from DR and potentially other sight threatening conditions. The ability of machine learning to detect or predict other medical comorbidities from retinal fundus photographs expands the possible applications beyond eye care alone (93). Further research is necessary to identify and address barriers to widespread adoption and better characterize the long-term impact on clinical outcomes and health care savings compared to in-person ophthalmic care.
Acknowledgments
Funding: None.
Footnote
Provenance and Peer Review: This article was commissioned by the Guest Editor (Kareem Moussa) for the series “The Retina and Systemic Disease” published in Annals of Eye Science. The article has undergone external peer review.
Reporting Checklist: The authors have completed the Narrative Review reporting checklist. Available at https://aes.amegroups.com/article/view/10.21037/aes-23-10/rc
Peer Review File: Available at https://aes.amegroups.com/article/view/10.21037/aes-23-10/prf
Conflicts of Interest: All authors have completed the ICMJE uniform disclosure form (available at https://aes.amegroups.com/article/view/10.21037/aes-23-10/coif). The series “The Retina and Systemic Disease” was commissioned by the editorial office without any funding or sponsorship. G.Y. received consulting fees from 4DMT, Abbvie, Adverum, Alimera, Bausch & Lomb, Boehringer Ingelheim, Clearside Biomedical, Endogena Therapeutics, Genentech, Gyroscope Therapeutics, Iridex, Janssen, jCyte, Myrobalan Therapeutics, NGM Biopharmaceutical, Novartis, Ocuphire, Ray BioTherapeutics, RegenXBio, West, Stealth Therapeutics. The authors have no other conflicts of interest to declare.
Ethical Statement: The authors are accountable for all aspects of the work in ensuring that questions related to the accuracy or integrity of any part of the work are appropriately investigated and resolved. All clinical procedures described in this study were performed in accordance with the ethical standards of the institutional and/or national research committee(s) and with the Helsinki Declaration (as revised in 2013). Written informed consent was obtained from the patient for the publication of this article and accompanying images.
Open Access Statement: This is an Open Access article distributed in accordance with the Creative Commons Attribution-NonCommercial-NoDerivs 4.0 International License (CC BY-NC-ND 4.0), which permits the non-commercial replication and distribution of the article with the strict proviso that no changes or edits are made and the original work is properly cited (including links to both the formal publication through the relevant DOI and the license). See: https://creativecommons.org/licenses/by-nc-nd/4.0/.
References
- Teo ZL, Tham YC, Yu M, et al. Global Prevalence of Diabetic Retinopathy and Projection of Burden through 2045: Systematic Review and Meta-analysis. Ophthalmology 2021;128:1580-91. [Crossref] [PubMed]
- Gange WS, Xu BY, Lung K, et al. Rates of Eye Care and Diabetic Eye Disease among Insured Patients with Newly Diagnosed Type 2 Diabetes. Ophthalmol Retina 2021;5:160-8. [Crossref] [PubMed]
- Sharma M, Jain N, Ranganathan S, et al. Tele-ophthalmology: Need of the hour. Indian J Ophthalmol 2020;68:1328-38. [Crossref] [PubMed]
- Grisolia ABD, Abalem MF, Lu Y, et al. Teleophthalmology: where are we now? Arq Bras Oftalmol 2017;80:401-6. [Crossref] [PubMed]
- Kalogeropoulos D, Kalogeropoulos C, Stefaniotou M, et al. The role of tele-ophthalmology in diabetic retinopathy screening. J Optom 2020;13:262-8. [Crossref] [PubMed]
- Liesenfeld B, Kohner E, Piehlmeier W, et al. A telemedical approach to the screening of diabetic retinopathy: digital fundus photography. Diabetes Care 2000;23:345-8. [Crossref] [PubMed]
- Surendran TS, Raman R. Teleophthalmology in Diabetic Retinopathy. J Diabetes Sci Technol 2014;8:262-6. [Crossref] [PubMed]
- Early Treatment Diabetic Retinopathy Study design and baseline patient characteristics. ETDRS report number 7. Ophthalmology 1991;98:741-56. [Crossref] [PubMed]
- Li HK, Horton M, Bursell SE, et al. Telehealth practice recommendations for diabetic retinopathy, second edition. Telemed J E Health 2011;17:814-37.
- Tozer K, Woodward MA, Newman-Casey PA. Telemedicine and Diabetic Retinopathy: Review of Published Screening Programs. J Endocrinol Diabetes 2015; [Crossref] [PubMed]
- Bouskill K, Smith-Morris C, Bresnick G, et al. Blind spots in telemedicine: a qualitative study of staff workarounds to resolve gaps in diabetes management. BMC Health Serv Res 2018;18:617. [Crossref] [PubMed]
- Abràmoff MD, Reinhardt JM, Russell SR, et al. Automated early detection of diabetic retinopathy. Ophthalmology 2010;117:1147-54. [Crossref] [PubMed]
- Abramoff MD, Suttorp-Schulten MS. Web-based screening for diabetic retinopathy in a primary care population: the EyeCheck project. Telemed J E Health 2005;11:668-74. [Crossref] [PubMed]
- Fenner BJ, Wong RLM, Lam WC, et al. Advances in Retinal Imaging and Applications in Diabetic Retinopathy Screening: A Review. Ophthalmol Ther 2018;7:333-46. [Crossref] [PubMed]
- Zimmer-Galler I, Zeimer R. Results of implementation of the DigiScope for diabetic retinopathy assessment in the primary care environment. Telemed J E Health 2006;12:89-98. [Crossref] [PubMed]
- Cuadros J, Bresnick G. EyePACS: an adaptable telemedicine system for diabetic retinopathy screening. J Diabetes Sci Technol 2009;3:509-16. [Crossref] [PubMed]
- Massin P, Chabouis A, Erginay A, et al. OPHDIAT: a telemedical network screening system for diabetic retinopathy in the Ile-de-France. Diabetes Metab 2008;34:227-34. [Crossref] [PubMed]
- Scanlon PH, Malhotra R, Thomas G, et al. The effectiveness of screening for diabetic retinopathy by digital imaging photography and technician ophthalmoscopy. Diabet Med 2003;20:467-74. [Crossref] [PubMed]
- Tennant MT, Rudnisky CJ, Hinz BJ, et al. Tele-ophthalmology via stereoscopic digital imaging: a pilot project. Diabetes Technol Ther 2000;2:583-7. [Crossref] [PubMed]
- Fonda SJ, Bursell SE, Lewis DG, et al. The Indian Health Service Primary Care-Based Teleophthalmology Program for Diabetic Eye Disease Surveillance and Management. Telemed J E Health 2020;26:1466-74. [Crossref] [PubMed]
- Lian JX, Gangwani RA, McGhee SM, et al. Systematic screening for diabetic retinopathy (DR) in Hong Kong: prevalence of DR and visual impairment among diabetic population. Br J Ophthalmol 2016;100:151-5. [Crossref] [PubMed]
- Sanchez CR, Silva PS, Cavallerano JD, et al. Ocular telemedicine for diabetic retinopathy and the Joslin Vision Network. Semin Ophthalmol 2010;25:218-24. [Crossref] [PubMed]
- Cavallerano AA, Cavallerano JD, Katalinic P, et al. A telemedicine program for diabetic retinopathy in a Veterans Affairs Medical Center--the Joslin Vision Network Eye Health Care Model. Am J Ophthalmol 2005;139:597-604. [Crossref] [PubMed]
- Conlin PR, Fisch BM, Cavallerano AA, et al. Nonmydriatic teleretinal imaging improves adherence to annual eye examinations in patients with diabetes. J Rehabil Res Dev 2006;43:733-40. [Crossref] [PubMed]
- Maa AY, Wojciechowski B, Hunt KJ, et al. Early Experience with Technology-Based Eye Care Services (TECS): A Novel Ophthalmologic Telemedicine Initiative. Ophthalmology 2017;124:539-46. [Crossref] [PubMed]
- Maa AY, Medert CM, Lu X, et al. Diagnostic Accuracy of Technology-based Eye Care Services: The Technology-based Eye Care Services Compare Trial Part I. Ophthalmology 2020;127:38-44. [Crossref] [PubMed]
- Wilson C, Horton M, Cavallerano J, et al. Addition of primary care-based retinal imaging technology to an existing eye care professional referral program increased the rate of surveillance and treatment of diabetic retinopathy. Diabetes Care 2005;28:318-22. [Crossref] [PubMed]
- Rudnisky CJ, Tennant MT, Weis E, et al. Web-based grading of compressed stereoscopic digital photography versus standard slide film photography for the diagnosis of diabetic retinopathy. Ophthalmology 2007;114:1748-54. [Crossref] [PubMed]
- Gibson DM. Frequency and predictors of missed visits to primary care and eye care providers for annually recommended diabetes preventive care services over a two-year period among U.S. adults with diabetes. Prev Med 2017;105:257-64. [Crossref] [PubMed]
- Shukla AK, Singh S, Sheikh A, et al. Diabetic retinopathy screening at primary and community health centers in Maharashtra. Indian J Ophthalmol 2020;68:S83-7. [Crossref] [PubMed]
- Law MG, Komura S, Murchison AP, et al. Pharmacy staff opinions regarding diabetic retinopathy screenings in the community setting: findings from a brief survey. Am Health Drug Benefits 2013;6:548-52. [PubMed]
- Phan RCV, Van Le D, Nguyen A, et al. Rapid Adoption of Telehealth at an Interprofessional Student-Run Free Clinic. PRiMER 2020;4:23. [Crossref] [PubMed]
- Okaka Y, Meah YS, Fallar R, et al. Ophthalmology Services at Student-Run Free Clinics: A National Survey. J Natl Med Assoc 2021;113:431-5. [Crossref] [PubMed]
- Rathi S, Tsui E, Mehta N, et al. The Current State of Teleophthalmology in the United States. Ophthalmology 2017;124:1729-34. [Crossref] [PubMed]
- Jani PD, Forbes L, Choudhury A, et al. Evaluation of Diabetic Retinal Screening and Factors for Ophthalmology Referral in a Telemedicine Network. JAMA Ophthalmol 2017;135:706-14. [Crossref] [PubMed]
- Safi S, Ahmadieh H, Katibeh M, et al. Modeling a Telemedicine Screening Program for Diabetic Retinopathy in Iran and Implementing a Pilot Project in Tehran Suburb. J Ophthalmol 2019;2019:2073679. [Crossref] [PubMed]
- Zikhali T, Kalinda C, Xulu-Kasaba ZN. Screening of Diabetic Retinopathy Using Teleophthalmology to Complement Human Resources for Eye Health: A Systematic Review and Meta-Analysis. Clin Pract 2022;12:457-67. [Crossref] [PubMed]
- Daskivich LP, Vasquez C, Martinez C Jr, et al. Implementation and Evaluation of a Large-Scale Teleretinal Diabetic Retinopathy Screening Program in the Los Angeles County Department of Health Services. JAMA Intern Med 2017;177:642-9. [Crossref] [PubMed]
- Scanlon PH. The English National Screening Programme for diabetic retinopathy 2003-2016. Acta Diabetol 2017;54:515-25. [Crossref] [PubMed]
- Lieng MK, Emami-Naeini P, Lee SC, et al. Teleophthalmology provides earlier eye care access for patients with newly-diagnosed diabetes. Heliyon 2024;10:e25845. [Crossref] [PubMed]
- Lee SC, Lieng MK, Alber S, et al. Trends in Remote Retinal Imaging Utilization and Payments in the United States. Ophthalmology 2022;129:354-7. [Crossref] [PubMed]
- Ellis MP, Bacorn C, Luu KY, et al. Cost Analysis of Teleophthalmology Screening for Diabetic Retinopathy Using Teleophthalmology Billing Codes. Ophthalmic Surg Lasers Imaging Retina 2020;51:S26-34. [Crossref] [PubMed]
- Lee SC, Alber S, Lieng MK, et al. Teleophthalmology Using Remote Retinal Imaging During the COVID-19 Pandemic. Telemed J E Health 2023;29:81-6. [Crossref] [PubMed]
- Ramasamy K, Mishra C, Kannan NB, et al. Telemedicine in diabetic retinopathy screening in India. Indian J Ophthalmol 2021;69:2977-86. [Crossref] [PubMed]
- Aruljyothi L, Bavishi A, Balasundaram MB, et al. Expanding the scope of tele-ophthalmology from vision centers to home. Indian J Ophthalmol 2021;69:442-6. [Crossref] [PubMed]
- Lu L, Ausayakhun S, Ausayakuhn S, et al. Diagnostic accuracy of handheld fundus photography: A comparative study of three commercially available cameras. PLOS Digit Health 2022;1:e0000131. [Crossref] [PubMed]
- Caceres J, Zhang Y, Boe L, et al. Diabetic Retinopathy Screening Using a Portable Retinal Camera in Vanuatu. Clin Ophthalmol 2023;17:2919-27. [Crossref] [PubMed]
- Tan CH, Kyaw BM, Smith H, et al. Use of Smartphones to Detect Diabetic Retinopathy: Scoping Review and Meta-Analysis of Diagnostic Test Accuracy Studies. J Med Internet Res 2020;22:e16658. [Crossref] [PubMed]
- Vujosevic S, Benetti E, Massignan F, et al. Screening for diabetic retinopathy: 1 and 3 nonmydriatic 45-degree digital fundus photographs vs 7 standard early treatment diabetic retinopathy study fields. Am J Ophthalmol 2009;148:111-8. [Crossref] [PubMed]
- Aiello LP, Odia I, Glassman AR, et al. Comparison of Early Treatment Diabetic Retinopathy Study Standard 7-Field Imaging With Ultrawide-Field Imaging for Determining Severity of Diabetic Retinopathy. JAMA Ophthalmol 2019;137:65-73. [Crossref] [PubMed]
- Ashraf M, Hock KM, Cavallerano JD, et al. Comparison of Widefield Laser Ophthalmoscopy and ETDRS Retinal Area for Diabetic Retinopathy. Ophthalmol Sci 2022;2:100190. [Crossref] [PubMed]
- Silva PS, Horton MB, Clary D, et al. Identification of Diabetic Retinopathy and Ungradable Image Rate with Ultrawide Field Imaging in a National Teleophthalmology Program. Ophthalmology 2016;123:1360-7. [Crossref] [PubMed]
- Silva PS, Cavallerano JD, Tolls D, et al. Potential efficiency benefits of nonmydriatic ultrawide field retinal imaging in an ocular telehealth diabetic retinopathy program. Diabetes Care 2014;37:50-5. [Crossref] [PubMed]
- Perrier M, Boucher MC, Angioi K, et al. Comparison of two, three and four 45 degrees image fields obtained with the Topcon CRW6 nonmydriatic camera for screening for diabetic retinopathy. Can J Ophthalmol 2003;38:569-74. [Crossref] [PubMed]
- Bragge P, Gruen RL, Chau M, et al. Screening for presence or absence of diabetic retinopathy: a meta-analysis. Arch Ophthalmol 2011;129:435-44. [Crossref] [PubMed]
- Abràmoff MD, Lavin PT, Birch M, et al. Pivotal trial of an autonomous AI-based diagnostic system for detection of diabetic retinopathy in primary care offices. NPJ Digit Med 2018;1:39. [Crossref] [PubMed]
- Grzybowski A, Brona P, Lim G, et al. Artificial intelligence for diabetic retinopathy screening: a review. Eye (Lond) 2020;34:451-60. [Crossref] [PubMed]
- Mehra AA, Softing A, Guner MK, et al. Diabetic Retinopathy Telemedicine Outcomes With Artificial Intelligence-Based Image Analysis, Reflex Dilation, and Image Overread. Am J Ophthalmol 2022;244:125-32. [Crossref] [PubMed]
- van der Heijden AA, Abramoff MD, Verbraak F, et al. Validation of automated screening for referable diabetic retinopathy with the IDx-DR device in the Hoorn Diabetes Care System. Acta Ophthalmol 2018;96:63-8. [Crossref] [PubMed]
- Rajalakshmi R, Subashini R, Anjana RM, et al. Automated diabetic retinopathy detection in smartphone-based fundus photography using artificial intelligence. Eye (Lond) 2018;32:1138-44. [Crossref] [PubMed]
- Bellemo V, Lim ZW, Lim G, et al. Artificial intelligence using deep learning to screen for referable and vision-threatening diabetic retinopathy in Africa: a clinical validation study. Lancet Digit Health 2019;1:e35-44. [Crossref] [PubMed]
- Gulshan V, Peng L, Coram M, et al. Development and Validation of a Deep Learning Algorithm for Detection of Diabetic Retinopathy in Retinal Fundus Photographs. JAMA 2016;316:2402-10. [Crossref] [PubMed]
- Raumviboonsuk P, Krause J, Chotcomwongse P, et al. Deep learning versus human graders for classifying diabetic retinopathy severity in a nationwide screening program. NPJ Digit Med 2019;2:25. [Crossref] [PubMed]
- Bora A, Balasubramanian S, Babenko B, et al. Predicting the risk of developing diabetic retinopathy using deep learning. Lancet Digit Health 2021;3:e10-9. [Crossref] [PubMed]
- Abràmoff MD, Folk JC, Han DP, et al. Automated analysis of retinal images for detection of referable diabetic retinopathy. JAMA Ophthalmol 2013;131:351-7. [Crossref] [PubMed]
- Abràmoff MD, Tobey D, Char DS. Lessons Learned About Autonomous AI: Finding a Safe, Efficacious, and Ethical Path Through the Development Process. Am J Ophthalmol 2020;214:134-42. [Crossref] [PubMed]
- Bhaskaranand M, Ramachandra C, Bhat S, et al. The Value of Automated Diabetic Retinopathy Screening with the EyeArt System: A Study of More Than 100,000 Consecutive Encounters from People with Diabetes. Diabetes Technol Ther 2019;21:635-43. [Crossref] [PubMed]
- Heydon P, Egan C, Bolter L, et al. Prospective evaluation of an artificial intelligence-enabled algorithm for automated diabetic retinopathy screening of 30 000 patients. Br J Ophthalmol 2021;105:723-8. [Crossref] [PubMed]
- Tufail A, Rudisill C, Egan C, et al. Automated Diabetic Retinopathy Image Assessment Software: Diagnostic Accuracy and Cost-Effectiveness Compared with Human Graders. Ophthalmology 2017;124:343-51. [Crossref] [PubMed]
- Ipp E, Liljenquist D, Bode B, et al. Pivotal Evaluation of an Artificial Intelligence System for Autonomous Detection of Referrable and Vision-Threatening Diabetic Retinopathy. JAMA Netw Open 2021;4:e2134254. [Crossref] [PubMed]
- Hacisoftaoglu RE, Karakaya M, Sallam AB. Deep Learning Frameworks for Diabetic Retinopathy Detection with Smartphone-based Retinal Imaging Systems. Pattern Recognit Lett 2020;135:409-17. [Crossref] [PubMed]
- Liu R, Wang X, Wu Q, et al. DeepDRiD: Diabetic Retinopathy-Grading and Image Quality Estimation Challenge. Patterns (N Y) 2022;3:100512. [Crossref] [PubMed]
- Benet D, Pellicer-Valero OJ. Artificial intelligence: the unstoppable revolution in ophthalmology. Surv Ophthalmol 2022;67:252-70. [Crossref] [PubMed]
- Liu X, Ali TK, Singh P, et al. Deep Learning to Detect OCT-derived Diabetic Macular Edema from Color Retinal Photographs: A Multicenter Validation Study. Ophthalmol Retina 2022;6:398-410. [Crossref] [PubMed]
- Varadarajan AV, Bavishi P, Ruamviboonsuk P, et al. Predicting optical coherence tomography-derived diabetic macular edema grades from fundus photographs using deep learning. Nat Commun 2020;11:130. [Crossref] [PubMed]
- Arcadu F, Benmansour F, Maunz A, et al. Deep learning algorithm predicts diabetic retinopathy progression in individual patients. NPJ Digit Med 2019;2:92. [Crossref] [PubMed]
- Ko J, Han J, Yoon J, et al. Assessing central serous chorioretinopathy with deep learning and multiple optical coherence tomography images. Sci Rep 2022;12:1831. [Crossref] [PubMed]
- Li Y, Feng W, Zhao X, et al. Development and validation of a deep learning system to screen vision-threatening conditions in high myopia using optical coherence tomography images. Br J Ophthalmol 2022;106:633-9. [Crossref] [PubMed]
- Ta AWA, Goh HL, Ang C, et al. Two Singapore public healthcare AI applications for national screening programs and other examples. Health Care Science 2022;1:41-57. [Crossref]
- Islam MM, Poly TN, Walther BA, et al. Artificial Intelligence in Ophthalmology: A Meta-Analysis of Deep Learning Models for Retinal Vessels Segmentation. J Clin Med 2020;9:1018. [Crossref] [PubMed]
- Xie Y, Nguyen QD, Hamzah H, et al. Artificial intelligence for teleophthalmology-based diabetic retinopathy screening in a national programme: an economic analysis modelling study. Lancet Digit Health 2020;2:e240-9. [Crossref] [PubMed]
- Ting DSW, Cheung CY, Lim G, et al. Development and Validation of a Deep Learning System for Diabetic Retinopathy and Related Eye Diseases Using Retinal Images From Multiethnic Populations With Diabetes. JAMA 2017;318:2211-23. [Crossref] [PubMed]
- Sayres R, Taly A, Rahimy E, et al. Using a Deep Learning Algorithm and Integrated Gradients Explanation to Assist Grading for Diabetic Retinopathy. Ophthalmology 2019;126:552-64. [Crossref] [PubMed]
- Krause J, Gulshan V, Rahimy E, et al. Grader Variability and the Importance of Reference Standards for Evaluating Machine Learning Models for Diabetic Retinopathy. Ophthalmology 2018;125:1264-72. [Crossref] [PubMed]
- Char DS, Shah NH, Magnus D. Implementing Machine Learning in Health Care - Addressing Ethical Challenges. N Engl J Med 2018;378:981-3. [Crossref] [PubMed]
- González-Gonzalo C, Thee EF, Klaver CCW, et al. Trustworthy AI: Closing the gap between development and integration of AI systems in ophthalmic practice. Prog Retin Eye Res 2022;90:101034. [Crossref] [PubMed]
- Evans NG, Wenner DM, Cohen IG, et al. Emerging Ethical Considerations for the Use of Artificial Intelligence in Ophthalmology. Ophthalmol Sci 2022;2:100141. [Crossref] [PubMed]
- Klarenbeek SE, Schuurbiers-Siebers OCJ, van den Heuvel MM, et al. Barriers and Facilitators for Implementation of a Computerized Clinical Decision Support System in Lung Cancer Multidisciplinary Team Meetings-A Qualitative Assessment. Biology (Basel) 2020;10:9. [Crossref] [PubMed]
- Morgenstern JD, Rosella LC, Daley MJ, et al. "AI's gonna have an impact on everything in society, so it has to have an impact on public health": a fundamental qualitative descriptive study of the implications of artificial intelligence for public health. BMC Public Health 2021;21:40. [Crossref] [PubMed]
- Liu TYA, Wu JH. The Ethical and Societal Considerations for the Rise of Artificial Intelligence and Big Data in Ophthalmology. Front Med (Lausanne) 2022;9:845522. [Crossref] [PubMed]
- Ramesh B, Kumar N. Towards Trustworthy Telemedicine: Applying Explainable AI for Remote Healthcare Recommendations. International Journal of Progressive Research in Engineering Management and Science 2023;3:258-65.
- Tom E, Keane PA, Blazes M, et al. Protecting Data Privacy in the Age of AI-Enabled Ophthalmology. Transl Vis Sci Technol 2020;9:36. [Crossref] [PubMed]
- Poplin R, Varadarajan AV, Blumer K, et al. Prediction of cardiovascular risk factors from retinal fundus photographs via deep learning. Nat Biomed Eng 2018;2:158-64. [Crossref] [PubMed]
- Ting DSW, Peng L, Varadarajan AV, et al. Deep learning in ophthalmology: The technical and clinical considerations. Prog Retin Eye Res 2019;72:100759. [Crossref] [PubMed]
- Sheng B, Chen X, Li T, et al. An overview of artificial intelligence in diabetic retinopathy and other ocular diseases. Front Public Health 2022;10:971943. [Crossref] [PubMed]
- Korot E, Pontikos N, Liu X, et al. Predicting sex from retinal fundus photographs using automated deep learning. Sci Rep 2021;11:10286. [Crossref] [PubMed]
- Gerke S, Babic B, Evgeniou T, et al. The need for a system view to regulate artificial intelligence/machine learning-based software as medical device. NPJ Digit Med 2020;3:53. [Crossref] [PubMed]
- Jin K, Ye J. Artificial intelligence and deep learning in ophthalmology: Current status and future perspectives. Adv Ophthalmol Pract Res 2022;2:100078. [Crossref] [PubMed]
- Abràmoff MD, Cunningham B, Patel B, et al. Foundational Considerations for Artificial Intelligence Using Ophthalmic Images. Ophthalmology 2022;129:e14-32. [Crossref] [PubMed]
- Yiu GC, Fong R, Mehta N. Reimbursement for Teleophthalmology for Remote Diabetic Eye Screening. Retinal Physician 2022. Available online: https://retinalphysician.com/issues/2022/januaryfebruary/reimbursement-for-teleophthalmology-for-remote-diabetic-eye-screening/
- Motozawa N, An G, Takagi S, et al. Optical Coherence Tomography-Based Deep-Learning Models for Classifying Normal and Age-Related Macular Degeneration and Exudative and Non-Exudative Age-Related Macular Degeneration Changes. Ophthalmol Ther 2019;8:527-39. [Crossref] [PubMed]
- Yellapragada B, Hornauer S, Snyder K, et al. Self-Supervised Feature Learning and Phenotyping for Assessing Age-Related Macular Degeneration Using Retinal Fundus Images. Ophthalmol Retina 2022;6:116-29. [Crossref] [PubMed]
- Gioia G, Salducci M. Medical and legal aspects of telemedicine in ophthalmology. Rom J Ophthalmol 2019;63:197-207. [Crossref] [PubMed]
- Nittari G, Khuman R, Baldoni S, et al. Telemedicine Practice: Review of the Current Ethical and Legal Challenges. Telemed J E Health 2020;26:1427-37. [Crossref] [PubMed]
- Mazzuca D, Borselli M, Gratteri S, et al. Applications and Current Medico-Legal Challenges of Telemedicine in Ophthalmology. Int J Environ Res Public Health 2022;19:5614. [Crossref] [PubMed]
- Fields BG. Regulatory, Legal, and Ethical Considerations of Telemedicine. Sleep Med Clin 2020;15:409-16. [Crossref] [PubMed]
- de Oliveira Andrade A, Soares AB, de Andrade Palis A, et al. On the use of telemedicine in the context of COVID-19: legal aspects and a systematic review of technology. Research on Biomedical Engineering 2022;38:209-27. [Crossref]
- Tan Y, Sun X. Ocular images-based artificial intelligence on systemic diseases. Biomed Eng Online 2023;22:49. [Crossref] [PubMed]
- Crigger E, Khoury C. Making Policy on Augmented Intelligence in Health Care. AMA J Ethics 2019;21:E188-191. [Crossref] [PubMed]
- Akinrinmade AO, Adebile TM, Ezuma-Ebong C, et al. Artificial Intelligence in Healthcare: Perception and Reality. Cureus 2023;15:e45594. [Crossref] [PubMed]
- Tinner L, Caldwell D, Campbell R. Community mobilisation approaches to preventing and reducing adolescent multiple risk behaviour: a realist review protocol. Syst Rev 2021;10:147. [Crossref] [PubMed]
Cite this article as: Chokshi T, Cruz MJ, Ross J, Yiu G. Advances in teleophthalmology and artificial intelligence for diabetic retinopathy screening: a narrative review. Ann Eye Sci 2024;9:9.