Longitudinal analysis of quantitative biomarkers using projection-resolved OCT angiography in retinal vein occlusion
Introduction
Retinal vein occlusion (RVO) is the second most common cause of retinal vascular disease worldwide after diabetic retinopathy, and its prevalence increases with age (1,2). Moreover, macular edema accounts for vision loss in well-perfused forms of RVO (3). The functional outcome in RVO patients is highly dependent on the presence/absence of macular edema, as well as of extensive nonperfusion/ischemia. Since it was introduced in the 1960s, fluorescein angiography (FA) has become the gold standard in detecting and evaluating retinal perfusion (or lack thereof) in RVO patients. Nevertheless, recent imaging modalities, such as optical coherence tomography angiography (OCTA), have become more and more widely used to assess retinal vascular disease qualitatively and quantitatively (3,4). In the particular case of RVO, several studies have focused on the qualitative analysis of OCTA images, demonstrating superficial and deep vascular complex microvascular changes, such as non-perfusion areas, vascular tortuosity, or collateral vessel formation, disruption of perifoveal capillary plexus, microaneurysms and cystoid spaces (5-7). Due to the inherent subjectivity of a qualitative assessment, recent studies have focused on potential quantitative biomarkers and their associations with disease severity. Among these quantitative variables, vascular density (VD) is the most widely used metric, readily available on several OCTA instruments. VD is dependent on vessel caliber, therefore skeletal density (SD) was introduced, as a variable representative of the length of the entire retinal vascular network, independent of vessel caliber (8). Other metrics, such as fractal dimension (FD) of central macular DVC has shown promise as a biomarker for peripheral nonperfusion in RVO (9). Fractals were first described by Benoit Mandelbrot in the 1967 and defined as self-similar patterns found in nature and biological systems (10), with the particularity of displaying the same pattern regardless of the level of magnification. Thus, the fractal analysis provides a different way of characterizing the branching geometry of the retinal vascular complex, in terms of complexity. All these quantitative biomarkers may provide an interesting way to assess treatment response in RVO patients, by quantifying the changes in complexity, homogeneity, and extension of the microvascular damage at the level of both retinal vascular complexes. However, while VD is available automatically, the computation of FD and SD needs a supplemental image processing step using various image analysis software. This study aims to evaluate a fully automated VD, SD and FD method for the longitudinal analysis of RVO eyes on OCTA images and to evaluate VD, SD, and FD as potential biomarkers for the visual outcome in these patients.
We present the following article in accordance with the MDAR reporting checklist (available at http://dx.doi.org/10.21037/aes-20-112).
Methods
Patients and data collection
Consecutive patients presenting with both treatment-naïve and previously treated RVO [both central retinal vein occlusion (CRVO) and branch retinal vein occlusion (BRVO)], which presented at the University Eye Clinic of Creteil between January 2017 and January 2019 were retrospectively included. All patients had undergone a comprehensive ophthalmological examination, including best-corrected visual acuity (BCVA) using an ETDRS scale, biomicroscopy, fluorescein angiography (FA), spectral-domain optical coherence tomography (SD-OCT), as well as OCTA on a 3 mm × 3 mm central area (10 degrees). SD-OCT and OCTA were also performed at the last follow up visit. Multimodal imaging, consisting of FA and SD-OCT, was used for a formal diagnosis of RVO at baseline for included patients.
Two distinct groups emerged from the initial RVO cohort: eyes with a diagnosis of CRVO were included in group 1, while eyes with BRVO were included in group 2. Moreover, group 3 consisted of control age-matched eyes.
Exclusion criteria were: diabetic retinopathy, previous retinal surgery, pathologic myopia, or ocular trauma. Patients with poor quality images on OCTA (signal strength index [SSI] lower than 60) or with important artifacts (due to eye movements or media opacities) were also excluded from this study. The study followed the tenets of the Declaration of Helsinki revised in 2013. This retrospective study was performed in accordance with French legislation; given that we have used existing, anonymized data, IRB was not mandatory. Patient consent was waived due to the retrospective nature of this study.
Image acquisition protocol for OCTA
OCTA is a novel imaging technique using the split spectrum amplitude-decorrelation angiography (SSADA) algorithm. Each acquisition is performed at a speed of 100 kHz, with an A-scan rate of 70,000 scans per second, using a light source centered on 840 nm and a bandwidth of 50 nm. Each OCTA volume contains 304×304 A-scans with two consecutive B-scans captured at each fixed position before proceeding to the next sampling location. Split-spectrum amplitude-decorrelation angiography was used to extract the OCTA information. Each OCTA volume is acquired in 3 seconds and two orthogonal OCTA volumes were acquired to perform motion correction to minimize motion artifacts arising from microsaccades and fixation changes. Angiography information displayed is the average of the decorrelation values when viewed perpendicularly through the thickness being evaluated. The machine software provides an automatic segmentation for the superficial and deep vascular complex (SVC, DVC). For included eyes, OCTA was performed using a 3 mm × 3 mm scanning area. Baseline OCTA imaging was compared to the final follow up visits OCTA. The projection artifact removal (PAR) algorithm embedded in the instrument was used to remove projection artifacts by removing the smaller peaks throughout the scan that represent shadows of the overlying vessels, based on the decorrelation value and logarithm of reflectance intensity. The details of this algorithm and its demonstration have already been published (11).
Quantitative image analysis: VD, SD, and FD
Using the embedded software in the OCTA instrument, automatically segmented images for the SVC and DVC at study inclusion and at last follow up were extracted in ‘PNG’ format. A custom, automated algorithm developed in Matlab (MATLAB and Statistics Toolbox Release 2017b, The MathWorks, Inc., Natick, Massachusetts, United States) was used to perform the quantitative analysis of the SVC and DVC. The grayscale images were then converted to binary images. All pixels in the input images with a luminance lower than 0.2 were replaced by pixels with the value 0 (black). The level value for binarization has been user-defined at 0.2. VD, defined as the number of white pixels/total number of pixels, was computed for each plexus on the respective resulting binary images, as well as a corresponding colormap. A skeletonized image was then generated automatically, by detecting the central axis of the white-pixelated microvasculature on the binary image, and SD was computed. FD was computed using the box-counting method on the skeletonized images for each plexus. To assess the robustness of our method, the resulting values for vascular density obtained by this custom automated algorithm were compared to the automatically generated software vascular density (AngioAnalytics™, AngioVue RTVue XR Avanti, Optovue, Inc., Freemont, CA, USA).
Statistical analysis
Statistical analysis was performed using STATA version 13.0. Qualitative variables were described in percentages and quantitative variables were described by their mean±standard deviation. The non-parametric Mann-Whitney test was used to compare the statistical distribution of the measured parameters and the paired-Wilcoxon test was used to compare measured parameters at baseline and the end of the follow-up. Bland Altman plots were generated to evaluate the agreement between the 2 measurement methods for VD (custom Matlab algorithm versus Angioanalytics). Logistic regression was used to determine the associations between follow up BCVA and baseline quantitative variables (VD, SD, FD). P<0.05 were retained as significant.
Results
Patient demographics and clinical data
21 consecutive eyes of 20 patients presenting with RVO (10 males, 10 females, mean age 67.38±13.44 years) and 20 healthy control eyes of 13 patients (5 males, 8 females, mean age 59.92±12.02 years) were included.
The final analysis was performed in 3 groups:
- Group 1 consisted of 13 eyes with CRVO (mean age 71.92±14.02 years, mean BCVA at baseline 0.43±0.32 logMAR, mean follow up 19.69±8.28 months; 8 out of 13 included eyes were treatment naïve at baseline, while 5 eyes had been previously treated by anti-angiogenic therapy.
- Group 2 consisted of 8 eyes with BRVO (mean age 62.78±10.83 years, mean BCVA at baseline 0.18±0.12 logMAR, mean follow up 26.12±10.26 months); 3 out of 8 eyes in this group were treatment-naïve at baseline, while 5 eyes had been previously treated by anti-angiogenic therapy;
- Group 3 consisted of 20 eyes of 13 healthy controls (mean age 59.92±12.02 years, mean BCVA was 0.10±0.02).
On the overall RVO cohort, the mean age was 67.38±13.44 years, with no statistically significant difference between the CRVO and the BRVO groups (P=0.10). Ten out of 21 RVO eyes had previously undergone anti-vascular endothelial growth factor (anti-VEGF) intravitreal injections. Of the previously treated eyes, 5 eyes (3 with CRVO and 2 with BRVO) had previously undergone peripheral laser photocoagulation.
Moreover, at baseline, 11 out of 13 eyes with CRVO (84.61%) included in group 1 presented with macular edema, with a mean central macular thickness (CMT) of 468±149.23 microns. In group 2, the baseline prevalence of macular edema was 77.77% (7 out of 8 eyes with BRVO), with a mean CMT of 374.89±62.17 microns.
During follow up, eyes in group 1 had undergone a mean of 11.2±3.3 intravitreal injections, while eyes in group 2 had undergone a mean of 7.31±2.1 intravitreal injections. At the last visit, 4 out of 13 (30.77%) eyes in group 1 had persistent macular edema, while only 1 out of 8 (11%) eyes included in group 2 presented with macular edema.
There were no statistically significant differences between the CRVO and the BRVO groups in the history of previous peripheral laser photocoagulation. The presence of macular collateral vessels was statistically associated with BRVO eyes (50% vs. 21.4% in CRVO, P=0.043). Clinical characteristics of included eyes are summarized in Table 1.
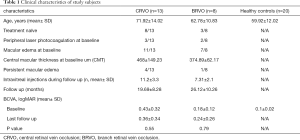
Full table
OCTA quantitative analysis
No statistically significant difference in terms of signal strength existed between groups 1, 2 and 3 (P=0.83).
Vascular density
In the overall cohort of patients presenting with RVO (21 eyes), VD at SVC level computed by AngioAnalytics was 39.66%±5.33% at baseline and decreased to 36.47%±3.91% at follow-up (P=0.002). When VD at SVC was computed with the custom Matlab algorithm it decreased from 39.78%±8.72% at baseline to 34.29%±6.67% at follow-up (P=0.0078). VD at DVC level remained stable over time, using the AngioAnalytics software: 40.08%±5.17% at baseline to 40.84%±6.18 at follow-up (P=0.96), as well as with our custom Matlab algorithm: 38.33%±7.53% to 38.53%±8.87% (P=0.79).
CRVO
In group 1, at baseline, mean VD using the embedded software (AngioAnalytics) averaged 37.63%±5.62% in the SVC and 39.01%±5.36% in the DVC. Using our custom algorithm, VD at baseline averaged 37.29%±9.38% in the SVC and 35.45%±6.57% in the DVC. At the last follow up visit in group 1, VD computed by using the embedded algorithm had decreased by 2.81%±2.11% in the SVC (P=0.036) and increased by 0.37%±4.70% in the DVC (P=0.88). Conversely, using our customized algorithm, VD decreased by 4.69%±8.92% in the SVC (P=0.075), while in the DVC the vascular density decreased by 0.68%±7.87% (P=0.92). Tables 2 and 3 summarize these findings.
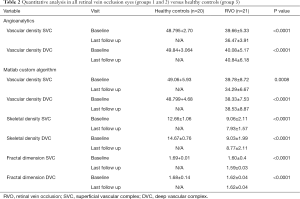
Full table
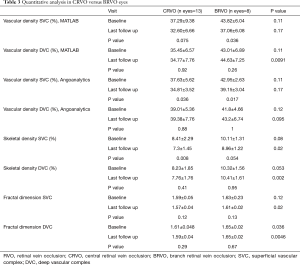
Full table
BRVO
Mean VD at baseline in group 2 using the embedded software (AngioAnalytics) averaged 42.95%±2.63% in the SVC and 41.8%±4.66 in the DVC. Using our custom algorithm, VD at baseline averaged 43.82%±6.04% in the SVC and 43.01%±6.89% in the DVC. At the last follow up visit in group 2, VD computed by using the embedded algorithm had decreased by 3.8%±3.19% in the SVC (P=0.017) and increased by 1.4%±8.31% in the DVC (P=1.00). Conversely, using our customized algorithm, VD decreased by 6.75%±6.25%, in the SVC (P=0.038) while in the DVC the vascular density decreased by 1.62%±8.90% (P=0.26).
Quantitative analysis using our custom algorithm, intergroup, and intragroup comparison using OCTA in both CRVO versus BRVO eyes is summarized in Table 2.
Group 3, consisting of healthy eyes, had an average VD at the level of the SVC of 48.79%±2.70% and 49.84%±3.06% at the level of the DVC, by using the embedded AngioAnalytics software. Conversely, using our custom algorithm, VD at baseline averaged 49.05%±5.93% in the SVC and 48.799%±4.68% in the DVC respectively.
Tables 2 and 3 summarize the quantitative analysis in RVO eyes (groups 1 and 2) versus healthy controls (group 3) using Angioanalytics versus our custom algorithm.
SD
In the overall cohort of patients, skeletal density (SD) at SVC decreased from 9.05%±2.11% at baseline to 7.93%±7.21% at follow-up (P=0.0087), while at DVC level SD decreased from 9.03%±1.99% at baseline to 8.77%±2.12% at follow-up (P=0.56). Comparison between SD in RVO groups and healthy controls is summarized in Table 2.
FD analysis
FD in the overall cohort of RVO patients slightly decreased over follow up at the level of SVC, from 1.60±0.04 at baseline to 1.59±0.03 at follow-up (P=0.04). At the level of the DVC, FD was stable in RVO eyes from 1.62±0.04 at baseline to 1.62±0.04 of the follow-up (P=0.44). Changes in FD over time in groups 1 and 2 are summarized in Table 2.
When comparing the CRVO group 1 and to the BRVO group 2, FD didn’t show statistically significant variations over time in the SVC (P=0.12 and P=0.13, respectively) or the DVC (P=0.29 and P=0.67, respectively). Instead, statistically significant differences between groups 1 and 2 were found in the SVC at the last follow-up visit (P=0.02) and in the DVC both at baseline and last follow-up (P=0.036 and P=0.0046, respectively).
Using our custom algorithm, for group 3 the baseline FD at the level of the SVC averaged 1.69±0.01 and 1.68±0.14 in the DVC. Figures 1-3 illustrate the SVC ans DVC analysis using VD, SD and FD with the custom algorithm. Figure 4 illustrates the box plot for FD values in the SVC and DVC in each group.
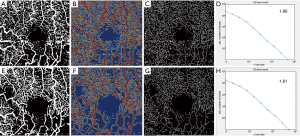
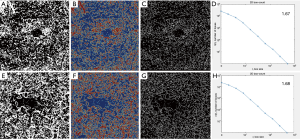
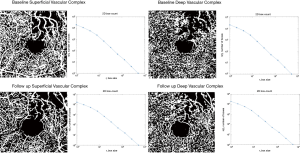
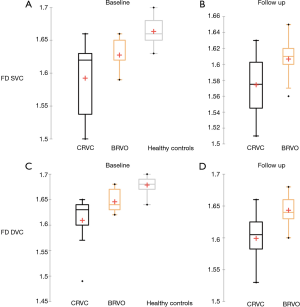
Biomarkers for visual outcomes
Logistic regression was computed to evaluate the correlation between baseline VD, FD, and SD and last visit BCVA in the overall cohort of RVO patients.
BCVA at follow-up was strongly correlated with the quantitative parameters evaluated at baseline at the level of SVC: VD [both computed with our custom Matlab algorithm (P=0.013) and computed with Angioanalytics (P=0.047), FD (P=0.016), as well as SD (P=0.01). Strongly correlated with final BCVA was also baseline FD at the DVC level (P=0.046) and FD at the DVC at last follow-up (P=0.025). Table 4 summarizes the results of the logistic regression.
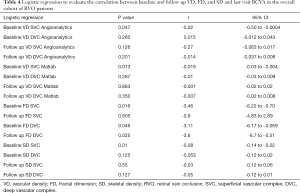
Full table
Reliability and agreement
Bland-Altman plot (Figure 5) was performed to compare the instrument’s embedded VD measurement to our custom algorithm. The agreement had a confidence interval [CI 95%] of 0.84 for VD on the overall cohort at the level of the SVC, while in the DVC the CI 95% was 0.71.
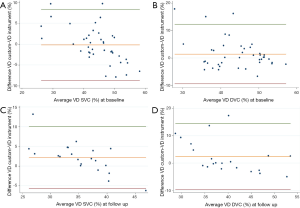
Discussion
In our study, OCTA images of the SVC and DVC were longitudinally analyzed in RVO patients with a fully automated algorithm, providing quantitative information about macular microvascular damage and suggesting predictive factors for the functional outcome in these patients. By designing a custom Matlab algorithm, we evaluated 3 possible quantitative biomarkers: VD, SD (reflecting vessel length and independent of vessel caliber), and FD (reflecting capillary complexity). First and foremost, we tested the reliability and agreement between the embedded software AngioAnalytics and our custom Matlab algorithm, showing a good confidence interval at both the level of the SVC and DVC.
When comparing RVO eyes to healthy controls, at the level of SVC, statistically significant lower values in terms of VD (obtained both with Angioanalytics and the Matlab custom algorithm) (P<0.0001 and P=0.0008 respectively) and FD (P<0.0001) were obtained. These results are consistent with the work of Koulisis et al. (12), that demonstrated significant differences in capillary density and morphology among subjects with BRVO and CRVO compared to controls. Moreover, we longitudinally analyzed RVO eyes after 2 years of follow-up. Our results showed that, at the level of the SVC, the evaluated quantitative parameters significantly decreased over time in all RVO eyes. VD decreased significantly in all the RVO eyes, both when it was computed automatically with Angioanalytics (P=0.002), as well as when the custom Matlab algorithm was used (P=0.0078) (Table 2). Moreover, VD of the SVC decreased overtime also when CRVO and BRVO groups were considered separately (Table 3). This progressive decrease in VD in RVO eyes is probably consequent to the extension of retinal non-perfusion in patients with RVO, a condition that has been described to characterize the conversion from nonischemic to ischemic RVO (13). Campochiaro et al. (14) demonstrated that a blockade of vascular endothelial growth factor (VEGF) by monthly anti-VEGF injections may reduce the progression of retinal nonperfusion at the posterior pole, but cannot prevent it. However, retinal nonperfusion may be present at the initial stage of the RVO and/or may appear only after treatment, with the regression of the cysts of macular edema (5).
Interestingly, all these quantitative parameters did not show a statistically significant decrease over time if computed at the level of the DVC (in all RVO eyes, P=0.79 for VD calculated with our custom Matlab algorithm, P=0.44 for FD, P=0.56 for SD). This may be an effect of anti-VEGF therapy. Indeed, Campochiaro et al. (14) reported that blockade of VEGF may not only prevent non-perfusion from worsening but also improve the retinal perfusion status. In a study by Suzuki et al. (15), anti-VEGF therapy reduced the non-perfusion areas and improved retinal flow, especially in the retinal DVC in RVO eyes. The authors explained that the densities of the capillaries were much greater in the DVC compared to the SVC, hence concluding that the DVC might be more susceptible to flow improvement after anti-VEGF therapy.
FD was shown to be a useful biomarker in the analysis of RVO patients. In a recent study, Cabral et al. showed that deep capillary plexus FD, VD, and lacunarity, describing the distribution of the gaps(lacunae) in a fractal image (16), were all associated with significant ischemia in RVO eyes, suggesting that fractal-based metrics of OCTA images may become a marker of ischemia in RVO (9). Our results showed that indeed the FD computed at the level of the DVC was the most reliable quantitative parameter in the differentiation of CRVO of BRVO. Indeed FD at DVC was statistically significantly lower in the CRVO group (group 1) than in the BRVO group (group 2) at baseline (P=0.036) and follow-up (P=0.0046). Nevertheless, at the level of the SVC, in terms of FD, the difference between the CRVO and BRVO groups was non-significant at baseline but revealed statistically lower values in the CRVO group at follow-up (P=0.02). According to our results, FD, especially at the DVC level, may progressively decrease, as the clinical severity of RVO increases (BRVO versus CRVO). This is consistent with other recent works. Coscas et al. first emphasized that microvascular damage, as assessed qualitatively, occurs predominantly in the DVC in RVO patients (6).
Koulisis et al., in a cross-sectional quantitative analysis of central macular microvasculature in RVO eyes, using OCTA, found a statistically significant difference in FD of all the vascular layers in subjects with BRVO and CRVO compared with controls and unaffected fellow eyes (12). In our study, the differences in terms of FD between CRVO and BRVO eyes are statistically significant, both at the SVC and DVC and even after almost 2 years of follow-up, showing how these vascular layers of different groups preserve their different pattern of complexity. FD, therefore, is a truthful biomarker of microvascular damage in RVO eyes.
To assess the association between these quantitative variables and the functional outcome (BCVA at last follow up) in the RVO patients, we used logistic regression. Our results showed that the variables which were correlated with the final BCVA in RVO eyes were baseline VD (Angioanalytics, P=0.047, Matlab, P=0.013), FD (P=0.016) and SD (P=0.01) at the level of the SVC. FD at the level of the DVC both at baseline and follow-up was also correlated with final BCVA (P=0.046, P=0.025 respectively). Hence, these variables may be predictive of the visual outcome in RVO patients and therefore may be considered as biomarkers.
To the best of our knowledge, this is the first work in which multiple quantitative variables are used to analyze RVO OCTA images longitudinally, and their potential as biomarkers for the functional outcome is assessed.
Our study has several limitations. Its small sample size and our instrument’s software segmentation errors due to the architectural disorganization, following macular edema, are the most important. Nevertheless, we were able to manually adjust the slab thickness and correct segmentation when needed. Moreover, the small scanning window (3 mm × 3 mm) allowed us the evaluation of the central 10 degrees in a very precise and detailed manner, but without assessing the peripheral retinal involvement.
In conclusion, the present study demonstrates that FD and SD, in addition to VD at baseline are associated with the functional outcome in RVO eyes. The corollary is that quantitative monitoring of the central macular microvascular damage in both edematous and ischemic RVO is of value, as it may shed light on the visual prognosis of RVO patients. This may help improve the evaluation and management of RVO patients.
Acknowledgments
Funding: None.
Footnote
Provenance and Peer Review: This article was commissioned by the Guest Editor (R Theodore Smith) for the series “Retinal Imaging and Diagnostics” published in Annals of Eye Science. The article has undergone external peer review.
Reporting Checklist: The authors have completed the MDAR reporting checklist. Available at http://dx.doi.org/10.21037/aes-20-112
Data Sharing Statement: Available at http://dx.doi.org/10.21037/aes-20-112
Conflicts of Interest: The authors have completed the ICMJE uniform disclosure form (available at http://dx.doi.org/10.21037/aes-20-112). The series “Retinal Imaging and Diagnostics” was commissioned by the editorial office without any funding or sponsorship. Dr. Miere reports personal fees from Allergan, personal fees from Novartis, personal fees from Optovue, personal fees from Bayer, outside the submitted work. The authors have no other conflicts of interest to declare.
Ethical Statement: The authors are accountable for all aspects of the work in ensuring that questions related to the accuracy or integrity of any part of the work are appropriately investigated and resolved. The study was conducted in accordance with the Declaration of Helsinki (as revised in 2013). The study used retrospective, anonymized data, therefore IRB was not mandatory and individual consent for this retrospective analysis was waived.
Open Access Statement: This is an Open Access article distributed in accordance with the Creative Commons Attribution-NonCommercial-NoDerivs 4.0 International License (CC BY-NC-ND 4.0), which permits the non-commercial replication and distribution of the article with the strict proviso that no changes or edits are made and the original work is properly cited (including links to both the formal publication through the relevant DOI and the license). See: https://creativecommons.org/licenses/by-nc-nd/4.0/.
References
- Rogers S, McIntosh RL, Cheung N, et al. The prevalence of retinal vein occlusion: pooled data from population studies from the United States, Europe, Asia, and Australia. Ophthalmology 2010;117:313-9.e1. [Crossref] [PubMed]
- Jaulim A, Ahmed B, Khanam T, et al. Branch retinal vein occlusion: epidemiology, pathogenesis, risk factors, clinical features, diagnosis, and complications. An update of the literature. Retina 2013;33:901-10. [Crossref] [PubMed]
- . Natural history and clinical management of central retinal vein occlusion. The Central Vein Occlusion Study Group. Arch Ophthalmol 1997;115:486-91. [Crossref] [PubMed]
- Jia Y, Bailey ST, Wilson DJ, et al. Quantitative optical coherence tomography angiography of choroidal neovascularization in age-related macular degeneration. Ophthalmology 2014;121:1435-44. [Crossref] [PubMed]
- Sellam A, Glacet-Bernard A, Coscas F, et al. Qualitative and quantitative follow-up using optical coherence tomography angiography of retinal vein occlusion treated with anti-VEGF: Optical Coherence Tomography Angiography Follow-up of Retinal Vein Occlusion. Retina 2017;37:1176-84. [Crossref] [PubMed]
- Coscas F, Glacet-Bernard A, Miere A, et al. Optical Coherence Tomography Angiography in Retinal Vein Occlusion: Evaluation of Superficial and Deep Capillary Plexa. Am J Ophthalmol 2016;161:160-71.e1. [Crossref] [PubMed]
- Tsai G, Banaee T, Conti FF, et al. Optical Coherence Tomography Angiography in Eyes with Retinal Vein Occlusion. J Ophthalmic Vis Res 2018;13:315-32. [Crossref] [PubMed]
- Kim AY, Rodger DC, Shahidzadeh A, et al. Quantifying Retinal Microvascular Changes in Uveitis Using Spectral-Domain Optical Coherence Tomography Angiography. Am J Ophthalmol 2016;171:101-12. [Crossref] [PubMed]
- Cabral D, Coscas F, Glacet-Bernard A, et al. Biomarkers of Peripheral Nonperfusion in Retinal Venous Occlusions Using Optical Coherence Tomography Angiography. Transl Vis Sci Technol 2019;8:7. [Crossref] [PubMed]
- Mandelbrot B. How long is the coast of britain? Statistical self-similarity and fractional dimension. Science 1967;156:636-8. [Crossref] [PubMed]
- Zhang M, Hwang TS, Campbell JP, et al. Projection-resolved optical coherence tomographic angiography. Biomed Opt Express 2016;7:816-28. [Crossref] [PubMed]
- Koulisis N, Kim AY, Chu Z, et al. Quantitative microvascular analysis of retinal venous occlusions by spectral domain optical coherence tomography angiography. PLoS One 2017;12:e0176404 [Crossref] [PubMed]
- Hayreh SS, Zimmerman MB, Podhajsky P. Incidence of various types of retinal vein occlusion and their recurrence and demographic characteristics. Am J Ophthalmol 1994;117:429-41. [Crossref] [PubMed]
- Campochiaro PA, Bhisitkul RB, Shapiro H, et al. Vascular endothelial growth factor promotes progressive retinal nonperfusion in patients with retinal vein occlusion. Ophthalmology 2013;120:795-802. [Crossref] [PubMed]
- Suzuki N, Hirano Y, Tomiyasu T, et al. Retinal Hemodynamics Seen on Optical Coherence Tomography Angiography Before and After Treatment of Retinal Vein Occlusion. Invest Ophthalmol Vis Sci 2016;57:5681-7. [Crossref] [PubMed]
- Gould DJ, Vadakkan TJ, Poché RA, Dickinson ME. Multifractal and lacunarity analysis of microvascular morphology and remodeling. Microcirculation. 2011;18:136-51. [Crossref] [PubMed]
Cite this article as: Miere A, Colantuono D, Jung C, Glacet-Bernard A, Becuwe S, Petit E, Souied E. Longitudinal analysis of quantitative biomarkers using projection-resolved OCT angiography in retinal vein occlusion. Ann Eye Sci 2021;6:14.